Author: Fabian Pedregosa <fabian.pedregosa@inria.fr>
FOSDEM 2011, Data Analytics Devroom
Outline
- What is scikits.learn ?
- Supervised, unsupervised learning
- Model selection
- Future directions
Introduction¶
scikits.learn is:
- General-purpose Python package for machine learning
- Easy to install: easy_install -U scikits.learn
- Consistent API, well documented
- Open source, BSD-licensed, community-driven project
Support Vector Machines¶
LibSVM on steroids¶
Efficient on both dense and sparse data: Faster and less memory usage on dense data.
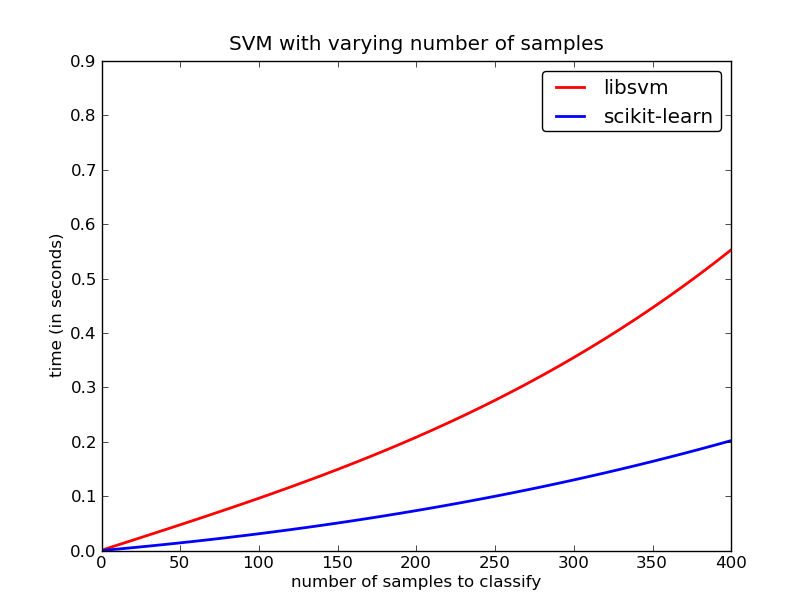
Weights on classes and samples
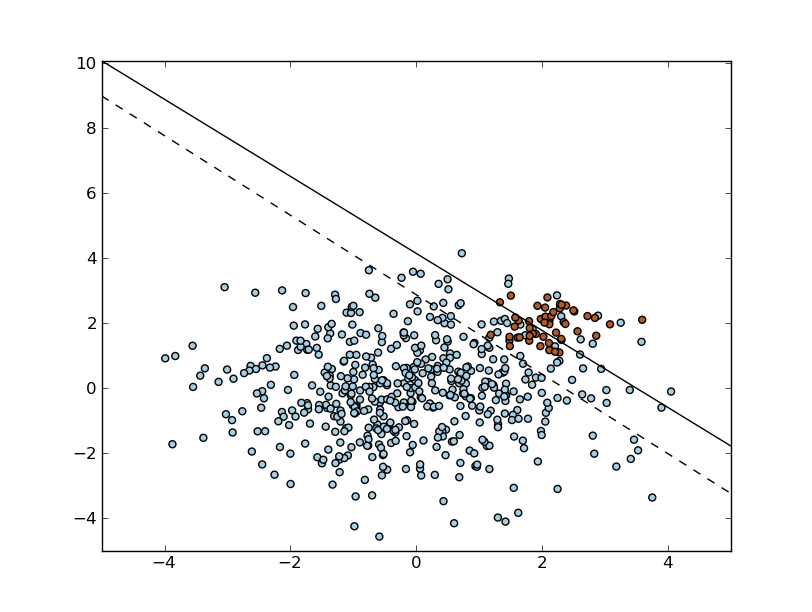
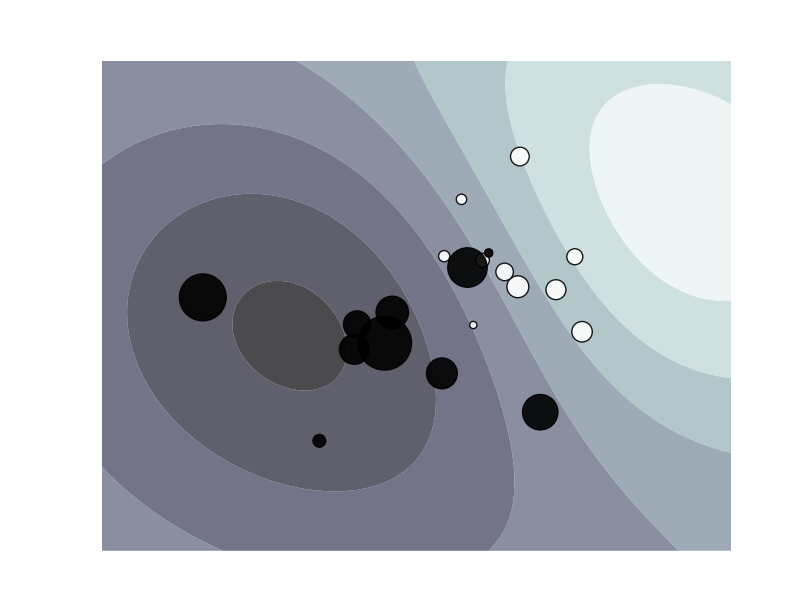
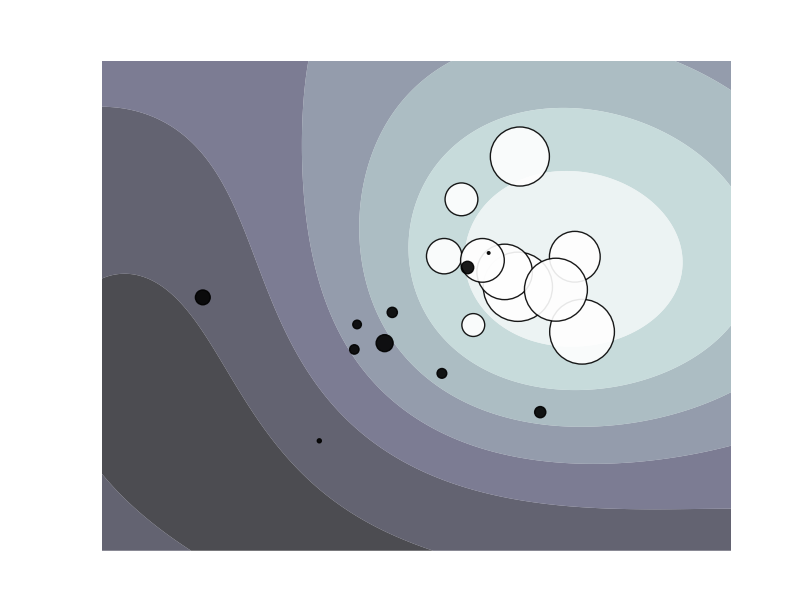
Different flavors: SVC, NuSVC, SVR, NuSVR, OneClass
LibLinear for large-scale learning: LinearSVC
Different kernels: Linear, Gaussian, Polynomial and custom
Custom kernels:
>>> import numpy as np
>>> from scikits.learn import svm
>>> def my_kernel(x, y):
... return np.dot(x, y.T)
...
>>> clf = svm.SVC(kernel=my_kernel)
Access to all parameters And indices of support vectors.
Linear Models¶
Lasso and ElasticNet¶
Lasso and ElasticNet are linear models with sparse (L1 and L1 + L2) regularization, and have become widely used in domains such as document classification, image deblurring, neuroimaging and genomics.
Two implementations for Lasso: by coordinate descent and by LARS, both state-of-the-art.
- LARS : gives the exact Lasso solution at the cost of a Least Squares.
- Coordinate descent : approximate method, extremely efficient in high-dimensional settings.
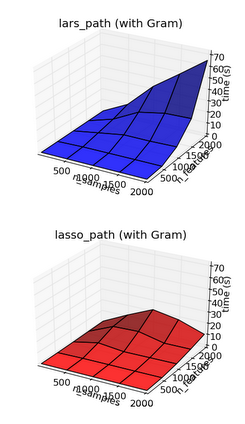
Large-scale learning¶
- Stochastic Gradient Descent
- LogisticRegression and LinearSVC using LibLinear
Benchmarks on a 500.000 sample dataset
Classifier | train-time | test-time |
---|---|---|
SVM (libsvm bindings) | >20min | |
LinearSVC (iblinear bindings) | 9.4471s | 0.0184s |
Stochastic Gradient Descent | 0.2137s | 0.0047s |
Unsupervised learning¶
RandomizedPCA, probabilistic version of PCA with better asymptotic properties.
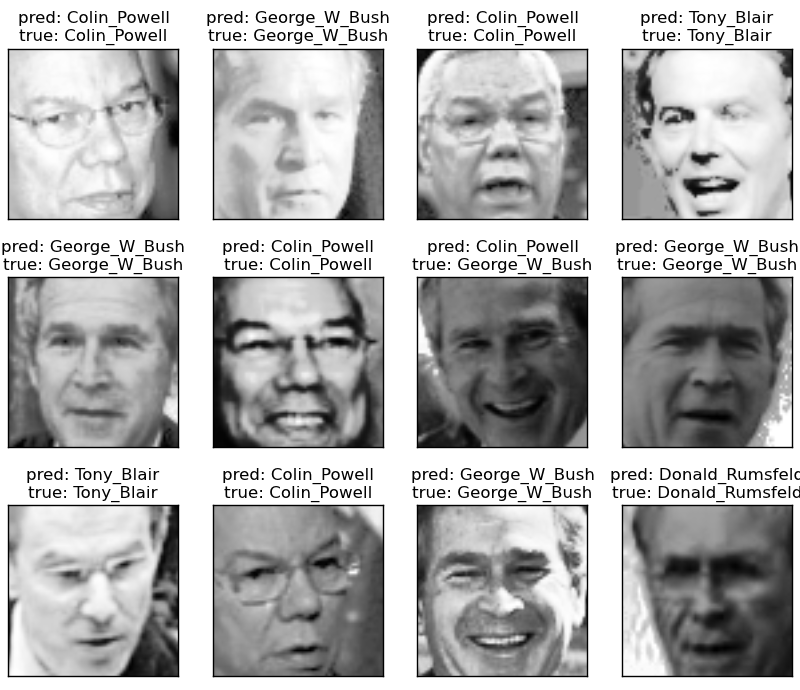
Clustering, GMM, etc.
Model Selection¶
GridSeachCV. Search optimal value by cross validation
>>> from scikits.learn import svm, grid_search, datasets
>>> iris = datasets.load_iris()
>>> param = {'C': np.arange(0.1, 2, 0.1)}
>>> svr = svm.SVR()
>>> clf = grid_search.GridSearchCV(svr, param)
>>> clf.fit(iris.data, iris.target)
GridSearchCV(n_jobs=1, fit_params={}, loss_func=None, iid=True,
estimator=SVR(kernel='rbf', C=1.0, probability=False, ...
in parallel!
However, this method is stupid and ignores all model specific information, thus some classes are able to automatically tune their parameters: LassoCV, ElasticNetCV, RidgeCV.
Statistics¶
- release each 2-3 months.
- 30 contributors (22 in the last release).
- Shipped with: Ubuntu, Debian, Macports, NetBSD, Mandriva, Enthought Python Distribution. Also easy_install and windows binaries.